Per cent difference or percentage difference is used to calculate how much two numbers vary between each other. It is presented as a percentage. Percentage difference is useful in manufacturing, design or engineering. Calculating the percentage difference between three numbers requires calculating the percentage differences between paired numbers of the three. Finding this result requires no mathematical knowledge beyond basic arithmetic. You will need knowledge of addition, averaging, division and how to convert a fraction to a percentage.
- Per cent difference or percentage difference is used to calculate how much two numbers vary between each other.
- You will need knowledge of addition, averaging, division and how to convert a fraction to a percentage.
Write down the three sums you are going to use for the problem. We'll use 3, 7 and 10 for this example.
Pick two of the numbers and subtract them from each other and write down the value. For example, subtracting 7 from 3 would result in an answer of -4. Remove any negative signs you may get as a result of your subtraction. This will leave you with a result of 4. Write this number down; you'll use it later in a division problem.
- Pick two of the numbers and subtract them from each other and write down the value.
- Write this number down; you'll use it later in a division problem.
Ignore the 4 for now and instead add the two number you originally picked. Adding 7 and 3 results in a sum of 10. Divide this number by 2 to find the average. The average here is 5.
Divide the difference from Step 2 by the average from Step 3, that is, 4 divided by 5 which results in .8. Use your calculator to solve the problem if necessary.
- Divide the difference from Step 2 by the average from Step 3, that is, 4 divided by 5 which results in .8.
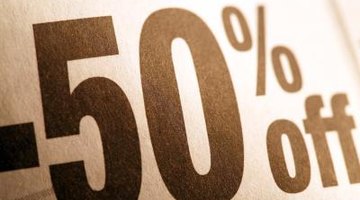
Multiple your result from Step 4 by 100 to get your percentage. The problem here would be .8 multiplied by 100. This results in 80. Write this number down on your paper and draw a percentage sign to the right. This is your percentage difference, which means there is an 80 per cent difference between 3 and 7.
Repeat this process by pairing up the rest of the numbers. For example, you'd solve the problems for the pair 3 and 10 and the pair 7 and 10. Write all your percentage differences down.